Image Super Resolution Using GAN
Introduction
In response to the growing demand for high-quality images, our team embarked on a project to enhance image resolution using Generative Adversarial Networks (GAN). The goal was to develop a model capable of significantly improving the clarity and details of low-resolution images.
Challenge
Low-resolution images often lack the necessary details for certain applications, such as image analysis, medical imaging, or content creation. The challenge was to create a solution that could generate high-resolution images from their low-resolution counterparts while maintaining realism and preserving essential features.
Solution
Our team implemented a GAN-based approach to tackle the image super-resolution problem. GANs consist of two neural networks – a generator and a discriminator – trained simultaneously in a competitive manner. The generator is responsible for creating high-resolution images, while the discriminator evaluates the generated images against real high-resolution images.
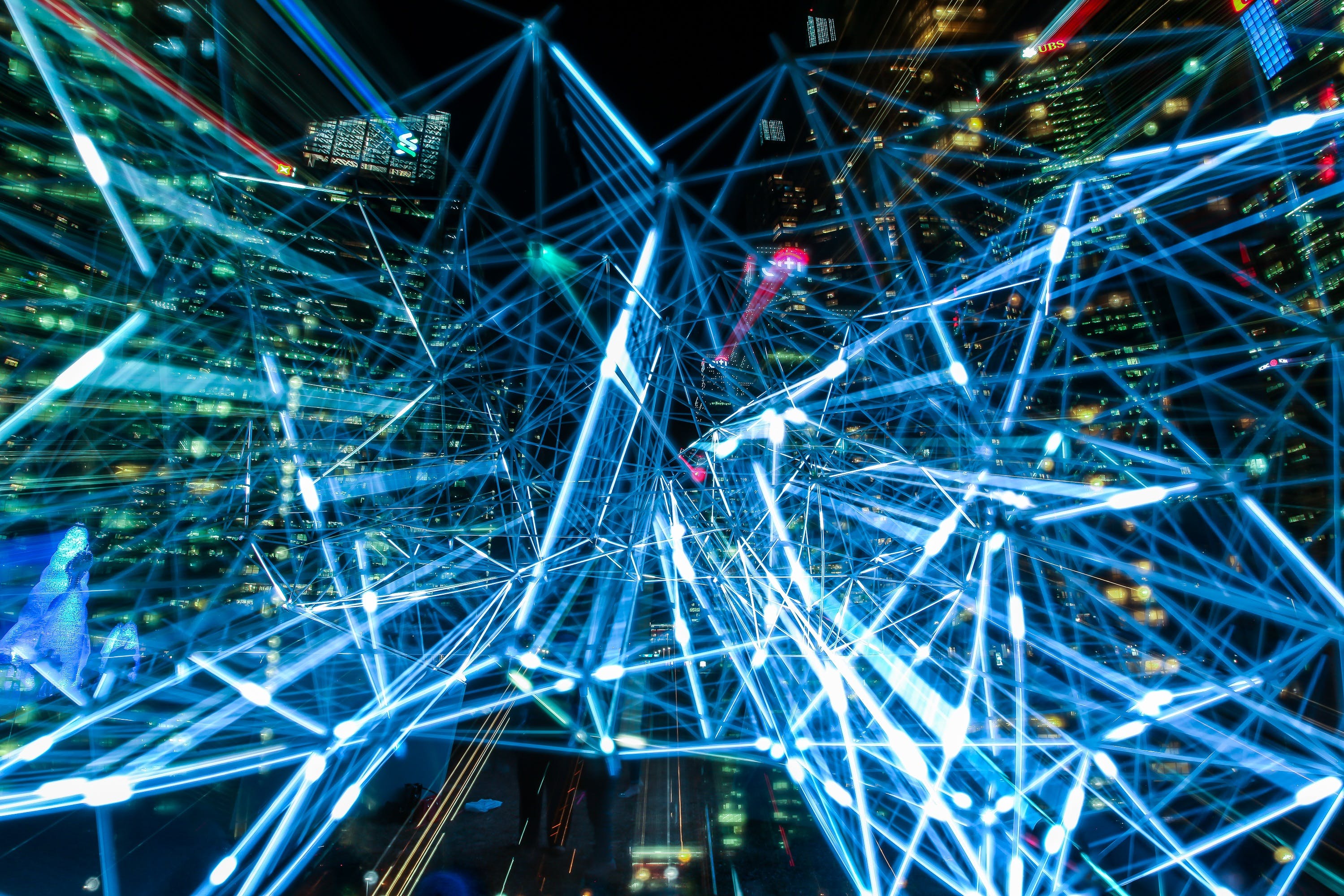
Implementation
1. Dataset Preparation
Curated a diverse dataset of low and high-resolution image pairs for training.
2. GAN Architecture
Designed a GAN architecture with a generator and discriminator using state-of-the-art techniques in deep learning.
3. Training Process
Trained the GAN on the dataset, optimizing the generator to produce high-resolution images that are indistinguishable from real high-resolution images.
4. Hyperparameter Tuning
Fine-tuned the model's hyperparameters to achieve optimal performance in terms of image quality and computational efficiency.
Results
The implementation of the GAN-based image super-resolution model yielded impressive results. The generated high-resolution images exhibited enhanced details and sharpness compared to their low-resolution counterparts. The model demonstrated its effectiveness across a variety of image types and performed well in real-world scenarios.
Quantifiable Outcomes
1. Improved Resolution
Achieved a significant increase in image resolution, making the enhanced images suitable for various applications requiring high-quality visuals.
2. Realism and Detail Preservation
Successfully maintained realism and preserved essential details during the super-resolution process.
3. Versatility
The model demonstrated versatility in handling diverse image types, from photographs to medical imaging scans.
Conclusion
The successful implementation of the GAN-based image super-resolution model underscores our commitment to leveraging innovative technologies to address real-world challenges. This project not only enhances our capabilities in image processing but also opens up new possibilities for industries relying on high-quality visual data.
Future Work
Continuing research and development in the field of image super-resolution, exploring applications in diverse industries, and staying at the forefront of advancements in GAN technology to further improve the performance of our models.
- Digital Creative Agency
- Professional Problem Solutions
- Web Design & Development